
In recent years, the limitations and shortcomings of the second generation HRA methods have led to further developments related to the improvement of pre-existing methods. The only method now defined as third generation is nuclear action reliability assessment (NARA) and is, in fact, an advanced version of HEART for the nuclear field. The shortcomings in the second generation, highlighted above, have been the starting point of HRA experts for new research and improvement of existing methods.
Some of the more recent studies have focused on lack of empirical data for development and validation of an HRA model and were intended to define the database HRA, which may provide the methodological tools needed to make greater use of more types of information in future HRAs and reduce uncertainties in the information used to conduct human reliability assessments. Currently, there are some databases for HRA analysts that contain the human error data with cited sources to improve the validity and reproducibility of HRA results. Examples of databases are the human event repository and analysis (HERA) 1 and the human factors information system (HFIS).
The PSFs are an integral part of the modelling and characterisation of errors and play an important role in the process of human reliability assessment; for this reason in recent years, HRA experts have focused their efforts on PSFs. Despite continuing advances in research and applications, one of the main weaknesses of current HRA methods is their limited ability to model the mutual influence among PSFs, intended both as a dependency among the states of the PSFs’ dependency among PSFs’ influences (impacts ) on human performance Figure 9.4 2 , 3 .
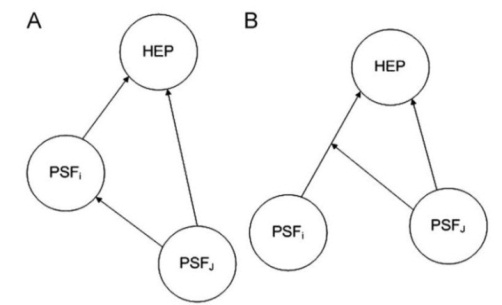
Some HRA methods – such as CREAM, SPAR-H, and IDAC – try to provide guidance on how to treat dependencies at the level of the factor assessments but do not consider that a PSF category might depend on itself and that the presence of a specific PSF might modulate the impact of another PSF on HEP; therefore, they do not adequately consider the relationships and dependencies between PSFs4 .Instead, De Ambroggi and Trucco’s (2011) study deals with the development of a framework for modelling the mutual influences existing among PSFs and a related method to assess the importance of each PSF in influencing performance of an operator, in a specific context, considering these interactions (see Figure 9.5).
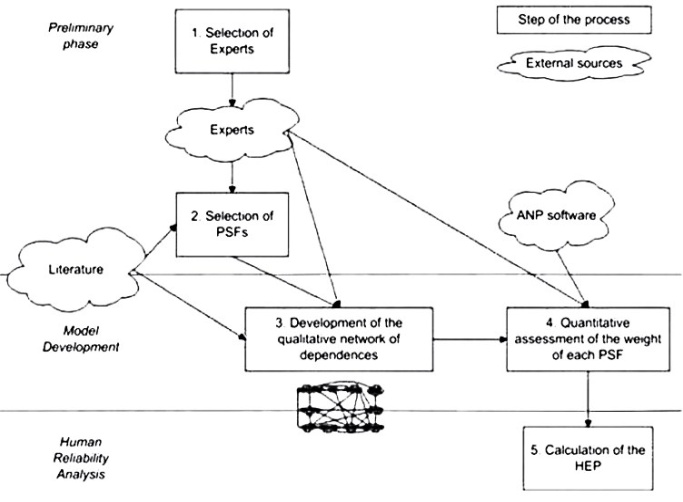
Another limitation of current HRA methods is the strong dependence on expert opinion to assign values to the PSFs; in fact, during this assignment process, subjectivity plays an important role, causing difficulties in assuring consistency. To overcome this problem and obtain a more precise estimation, Park and Lee (2008) suggest a new and simple method: AHP–SLIM5. This method combines the decision-making tool AHP – a multicriteria decision method for complex problems in which both qualitative and quantitative aspects are considered to provide objective and realistic results – with success likelihood index method (SLIM), a simple, flexible method of the expert judgement for estimating HEPs6, 7. Therefore through a type of HEP estimation using an analytic hierarchy process (AHP), it is possible to quantify the subjective judgement and confirm the consistency of collected data (see Figure 9.6).
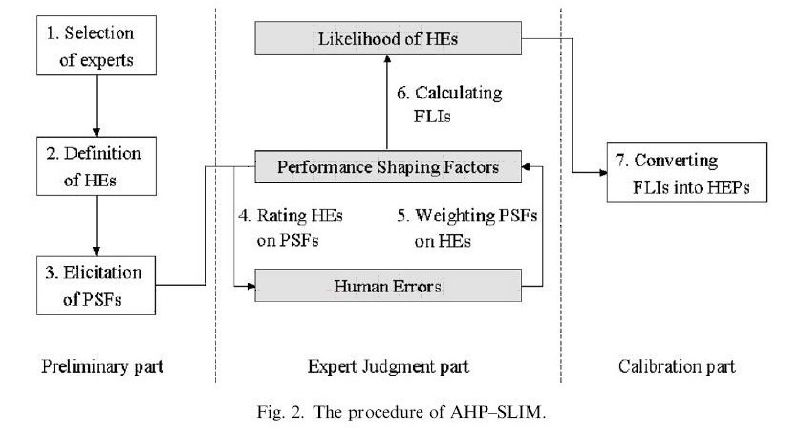
The real development concerns, however, are the so-called methods of reliability dynamics. Cacciabue8outlined the importance of simulation and modelling of human performance for the field of HRA. Specifically, simulation and modelling address the dynamic nature of human performance in a way not found in most HRA methods9. A cognitive simulation consists of the reproduction of a cognition model using a numerical application or computation 10, 11 .
As depicted in Figure 9.7, simulation and modelling may be used in three ways to capture and generate data that are meaningful to HRA12:
- The simulation runs produce logs, which may be analysed by experts and used to inform an estimate of the likelihood of human error;
- The simulation may be used to produce estimates PSFs, which can be quantified to produce human error probabilities (HEPs);
- A final approach is to set specific performance criteria by which the virtual performers in the simulation are able to succeed or fail at given tasks. Through iterations of the task that systematically explore the range of human performance, it is possible to arrive at a frequency of failure (or success). This number may be used as a frequentist approximation of an HEP.
Concurrent to the emergence of simulation and modelling, several authors (e.g. Jae and Park 1994; Sträter 2000) have posited the need for dynamic HRA and begun developing new HRA methods or modifying existing HRA methods to account for the dynamic progression of human behaviour leading up to and following human failure events (HFEs) 13.There is still not a tool for modelling and simulation that fully or perfectly combines all the basic elements of simulation HRA. There is, however, a significant work in progress, as for the simulator PROCOS, developed by Trucco and Leva in 2006 or for the IDAC system, which combines a realistic plant simulator with a system of cognitive simulation capable of modelling the PSF. In addition to systems such as MIDAS, in which the modelling of the error was already present, further efforts are to instill the PSF of SPAR-H in the simulation system14. PROCOS15, 16 is a probabilistic cognitive simulator for HRA studies, developed to support the analysis of human reliability in operational contexts complex. The simulation model comprised two cognitive flow charts, reproducing the behaviour of a process industry operator. The aim is to integrate the quantification capabilities of HRA methods with a cognitive evaluation of the operator (see Figure 9.8).
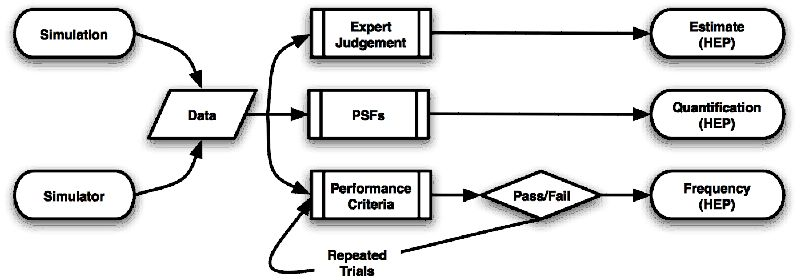
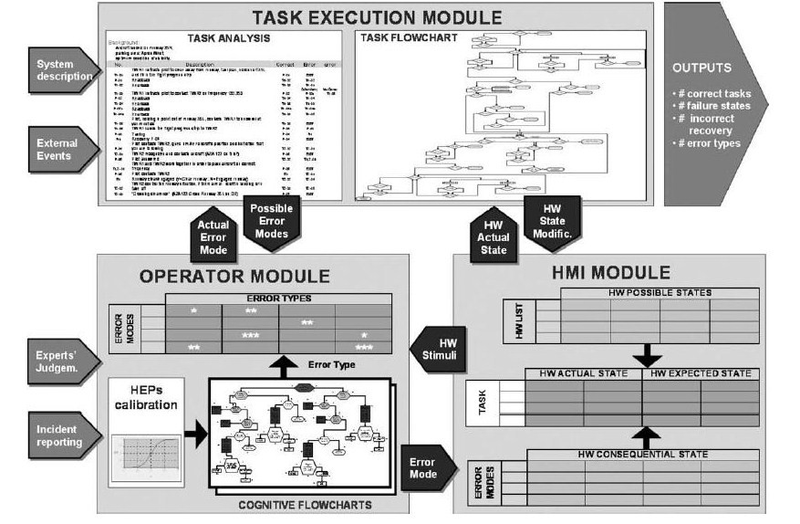
The model used for the configuration of the flow diagram that represents the operators is based on a combination of PIPE and SHELL. The two combined models allow for representation of the main cognitive processes that an operator can carry out to perform an action (PIPE) and describe the interaction among procedures, equipment, environment and plants present in the working environment, and the operator, as well as taking into account the possibility of interaction of the operator with other operators or supervisors (SHELL).
The IDAC model17; 18; 19; 20; 21; 22 is an operator behaviour model developed based on many relevant findings from cognitive psychology, behavioural science, neuroscience, human factors, field observations, and various first and second generation HRA approaches. In modelling cognition, IDAC combines the effects of rational and emotional dimensions (within the limited scope of modelling the behaviour of operators in a constrained environment) through a small number of generic rules-of-behaviour that govern the dynamic responses of the operator. The model constrained behaviour, largely regulated through training, procedures, standardised work processed, and professional discipline. This significantly reduces the complexity of the problem, as compared to modelling general human response. IDAC covers the operator’s various dynamic response phases, including situation assessment, diagnosis, and recovery actions in dealing with an abnormal situation. At a high level of abstraction, IDAC is composed of models of information processing (I), problem-solving and decision-making (D), and action execution (A) of a crew (C). Given incoming information, the crew model generated a probabilistic response, linking the context to the action through explicit causal chains. Due to the variety, quantity, and details of the input information, as well as the complexity of applying its internal rules, the IDAC model can only be presently implemented through a computer simulation (see Figure 9.9).
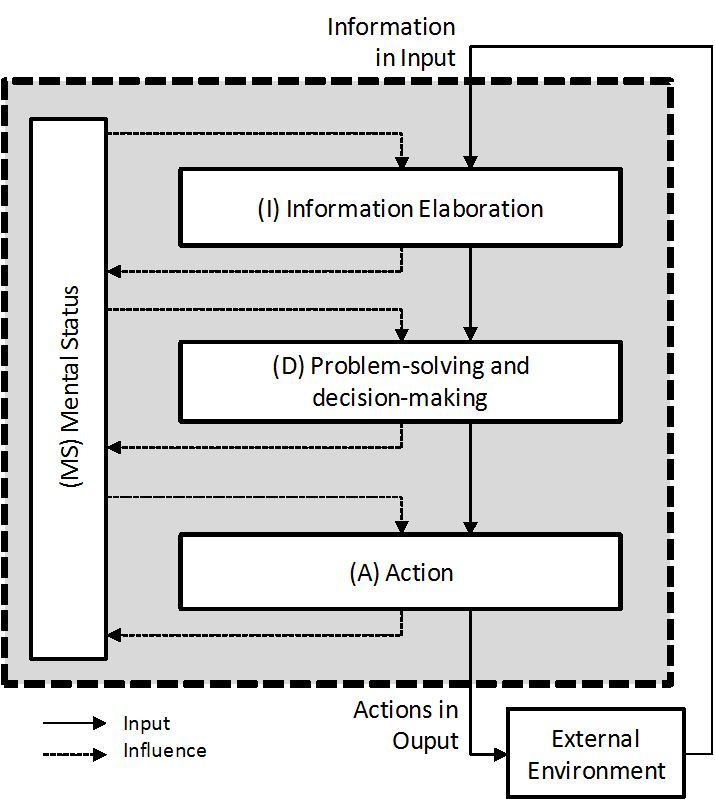
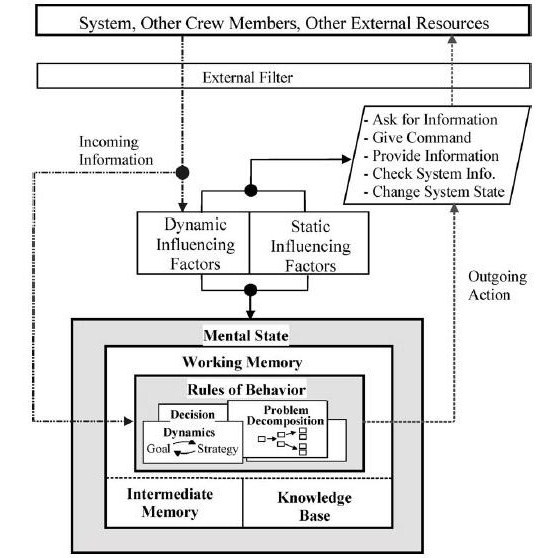
- 1704 reads